RFID en la Industria 4.0
El Papel de la Tecnología en la Operacionalización del Control de Planta
DOI:
https://doi.org/10.23925/2179-3565.2022v13i4p174-185Palabras clave:
Industria 4.0, RFID, Planificacion y Control de la Fabricación, Control de Piso de Fabrica, Revisión Sistemática de la LiteraturaResumen
El advenimiento de las tecnologías de la información y la comunicación ha trído muchos avances en el campo de la gestión de operaciones. RFID, cuando se utiliza como un sistema de coordinación de pedidos, brinda beneficios no solo para el control de producción sino también para varias funciones organizativas de apoyo a la fabricación. Para identificar estos beneficios, este trabajó utilizó una revisión sistemática de la literatura. Los resultados mostraron 15 beneficios, classificados en 4 áreas (funciones organizacionales) que impactam el control de planta y un modelo teórico que contiene el flujo de información necesario para operacionalizar el control de planta autónomo (4.0). La discusión presentó varias percepciones relacionando los beneficios com el índice de madurez de todo el ecosistema que conforma el control de la planta cuando adopta la tecnología RFID como Sistema de Coordinación de pedidos y una breve agenda de futuras investigaciones en este campo del conocimento. Se pudo concluir que, si bien el uso de la tecnología RFID no es algo nuevo, su uso como premisa para la operacionalización del control de planta aún se encuentra en etapas tempranas de madurez, lo que puede verse reforzado por el hecho de que se han identificado muchos beneficios asociado con las etapas iniciales del flujo de información presentado en el modelo teórico.
Citas
Altaf, M. S., Bouferguene, A., Liu, H., Al-Hussein, M., & Yu, H. (2018). Integrated production planning and control system for a panelized home prefabrication facility using simulation and RFID. Automation in Construction, 85(August 2017), 369–383. https://doi.org/10.1016/j.autcon.2017.09.009
Arena, M., Di Pasquale, V., Iannone, R., Miranda, S., & Riemma, S. (2022). A maintenance driven scheduling cockpit for integrated production and maintenance operation schedule. Advances in Manufacturing. https://doi.org/10.1007/s40436-021-00380-z
Arena, S., Florian, E., Zennaro, I., Orrù, P. F., & Sgarbossa, F. (2022). A novel decision support system for managing predictive maintenance strategies based on machine learning approaches. Safety Science, 146(October 2021), 105529. https://doi.org/10.1016/j.ssci.2021.105529
Bauer, A., Browne, J., Bowden, R., & Duggan, J. (1994). Shop Floor Control: From Design to Implementation (S. S. and B. Media (ed.)).
Bayano-Tejero, S., Sola-Guirado, R. R., Gil-Ribes, J. A., & Blanco-Roldan, G. L. (2019). Machine to machine connections for integral management of the olive production. Computers and Electronics in Agriculture, 166.
Chang, R. I., Lee, C. Y., & Hung, Y. H. (2021). Cloud-based analytics module for predictive maintenance of the textile manufacturing process. Applied Sciences (Switzerland), 11(21). https://doi.org/10.3390/app11219945
Christou, I. T., Kefalakis, N., Soldatos, J. K., & Despotopoulou, A. M. (2022). End-to-end industrial IoT platform for Quality 4.0 applications. Computers in Industry, 137, 103591. https://doi.org/10.1016/j.compind.2021.103591
Denyer, D., & Tranfield, D. (2009). Producing a Systematic Review. In The SAGE Handbook of Organizational Research Methods (pp. 671–689). https://doi.org/10.1080/03634528709378635
Dinesh, M., Arvind, C., Sreeja Mole, S. S., Subash Kumar, C. S., Chandra Sekar, P., Somasundaram, K., Srihari, K., Chandragandhi, S., & Sundramurthy, V. P. (2022). An Energy Efficient Architecture for Furnace Monitor and Control in Foundry Based on Industry 4.0 Using IoT. Scientific Programming, 2022, 1–8. https://doi.org/10.1155/2022/1128717
Fan, S. K. S., Hsu, C. Y., Tsai, D. M., Chou, M. C., Jen, C. H., & Tsou, J. H. (2022). Key Feature Identification for Monitoring Wafer-to-Wafer Variation in Semiconductor Manufacturing. IEEE Transactions on Automation Science and Engineering, 1–12. https://doi.org/10.1109/TASE.2022.3141426
Fang, J., Huang, G. Q., & Li, Z. (2013). Event-driven multi-agent ubiquitous manufacturing execution platform for shop floor work-in-progress management. International Journal of Production Research, 51(4), 1168–1185. https://doi.org/10.1080/00207543.2012.693644
Fang, J., Huang, G. Q., Qu, T., & Zhang, Y. F. (2011). RFID-enabled complex event processing application framework for manufacturing. International Journal of Services Operations and Informatics, 6(1–2), 30–44. https://doi.org/10.1504/IJSOI.2011.038313
Gartner Glossary. (n.d.).
Ghaleb, M., Taghipour, S., & Zolfagharinia, H. (2021). Real-time integrated production-scheduling and maintenance-planning in a flexible job shop with machine deterioration and condition-based maintenance. Journal of Manufacturing Systems, 61, 423–449.
Haghnegahdar, L., Joshi, S. S., & Dahotre, N. B. (2022). From IoT-based cloud manufacturing approach to intelligent additive manufacturing: industrial Internet of Things—an overview. International Journal of Advanced Manufacturing Technology, 119(3–4), 1461–1478. https://doi.org/10.1007/s00170-021-08436-x
Han, G., Tu, J., Liu, L., Martinez-Garcia, M., & Choi, C. (2022). An Intelligent Signal Processing Data Denoising Method for Control Systems Protection in the Industrial Internet of Things. IEEE Transactions on Industrial Informatics, 18(4), 2684–2692.
Hoffmann Souza, M. L., da Costa, C. A., de Oliveira Ramos, G., & da Rosa Righi, R. (2021). A feature identification method to explain anomalies in condition monitoring. Computers in Industry, 133.
Kanagachidambaresan, G. R. et. al. (2020). Internet of Things for Industry 4.0 Design, Challenges and Solutions.
Kibira, D., Morris, K. C., & Kumaraguru, S. (2016). Methods and tools for performance assurance of smart manufacturing systems. Journal of Research of the National Institute of Standards and Technology, 121, 282–313. https://doi.org/10.6028/jres.121.013
Kuftinova, N. G., Ostroukh, A. V., Maksimychev, O. I., Vasil’ev, Y. E., & Klimenko, V. A. (2022). Digital Twins in Smart Data Management at a Manufacturing Enterprise. Russian Engineering Research, 42(2), 162–164. https://doi.org/10.3103/S1068798X22020149
Kuftinova, N. G., Ostroukh, A. V., Maksimychev, O. I., Vasil’ev, Y. E., & Pletnev, M. G. (2022). Predictive Diagnostics and Maintenance of Industrial Equipment. Russian Engineering Research, 42(2), 158–161. https://doi.org/10.3103/S1068798X22020137
Lam, J. (2003). Enterprises Risk Management: From Incentives to Control (181st ed.). Jhon Wiley & Sons.
Lee, C. K. H., Ho, G. T. S., Choy, K. L., & Pang, G. K. H. (2014). A RFID-based recursive process mining system for quality assurance in the garment industry. International Journal of Production Research, 52(14), 4216–4238.
Lee, H. J., Lee, S., & Lee, J. M. (2022). Online Synchronization in Latent Variable Model Predictive Control for Trajectory Tracking of an Uneven Batch Process. Industrial and Engineering Chemistry Research, 61(1), 594–604. https://doi.org/10.1021/acs.iecr.1c03898
Lee, J. D., Hsu, H. Y., Li, C. Y., & Yang, J. Y. (2021). Design and implementation of intelligent automated production-line control system. Electronics (Switzerland), 10(20). https://doi.org/10.3390/electronics10202502
Li, X., Du, B., Li, Y., & Zhuang, K. (2019). RFID-based tracking and monitoring approach of real-time data in production workshop. Assembly Automation, 39(4), 648–663. https://doi.org/10.1108/AA-06-2018-080
Liu, C., Li, H., Tang, Y., Lin, D., & Liu, J. (2019). Next generation integrated smart manufacturing based on big data analytics, reinforced learning, and optimal routes planning methods. International Journal of Computer Integrated Manufacturing, 32(9), 820–831. https://doi.org/10.1080/0951192X.2019.1636412
Liu, J., Ma, C., Gui, H., & Wang, S. (2022). A four-terminal-architecture cloud-edge-based digital twin system for thermal error control of key machining equipment in production lines. Mechanical Systems and Signal Processing, 166(October 2021), 108488. https://doi.org/10.1016/j.ymssp.2021.108488
Liu, Y., Zhang, J., Hu, X., & Sun, S. (2022). Sensor data anomaly detection and correction for improving the life prediction of cutting tools in the slot milling process. International Journal of Advanced Manufacturing Technology, 119(1–2), 463–475. https://doi.org/10.1007/s00170-021-08275-w
Long, J. (2022). Dynamic Analysis of Alternative Elements in an Automated Packaging System Based on 5G Internet of Things. Journal of Sensors, 2022. https://doi.org/10.1155/2022/4909942
Mendia, I., Gil-López, S., Landa-Torres, I., Orbe, L., & Maqueda, E. (2022). Machine learning based adaptive soft sensor for flash point inference in a refinery realtime process. Results in Engineering, 13. https://doi.org/10.1016/j.rineng.2022.100362
Meng, Z., Wu, Z., & Gray, J. (2019). RFID-Based Object-Centric Data Management Framework for Smart Manufacturing Applications. IEEE INTERNET OF THINGS JOURNAL, 6(2), 2706–2716. https://doi.org/10.1109/JIOT.2018.2873426
Oluyisola, O. E., Bhalla, S., Sgarbossa, F., & Strandhagen, J. O. (2022). Designing and developing smart production planning and control systems in the industry 4.0 era: a methodology and case study. Journal of Intelligent Manufacturing, 33(1), 311–332. https://doi.org/10.1007/s10845-021-01808-w
Oluyisola, O. E., Sgarbossa, F., & Strandhagen, J. O. (2020). Smart production planning and control: Concept, use-cases and sustainability implications. Sustainability (Switzerland), 12(9). https://doi.org/10.3390/su12093791
PISSARDINI, P. E., & SACOMANO, J. B. (2019). Sistema de Coordenação de Ordens para a Indústria 4.0: Proposta de uma Arquitetura para implantação. “Os Desafios Da Engenharia de Produção Para Uma Gestão Inovadora Da Logística e Operações, 0–13. https://doi.org/10.14488/enegep2019_tn_wpg_290_635_36983
Poon, T. C., Choy, K. L., & Lau, H. C. W. (2007). A real-time shop floor control system: an integrated RFID approach. International Journal of Enterprise Network Management, 1(4), 331–349. https://doi.org/10.1504/IJENM.2007.013903
Qian, H., Sun, B., Guo, Y., Yang, Z., Ling, J., & Feng, W. (2022). A parallel deep learning algorithm with applications in process monitoring and fault prediction. Computers and Electrical Engineering, 99.
Saygin, C., & Sarangapani, J. (2006). RFID on the manufacturing shop floor: Applications and challenges. 2006 IIE Annual Conference and Exhibition, 2.
Schäfers, P., Mütze, A., & Nyhuis, P. (2019). Integrated Concept for Acquisition and Utilization of Production Feedback Data to Support Production Planning and Control in the Age of Digitalization. Procedia Manufacturing, 31, 225–231. https://doi.org/10.1016/j.promfg.2019.03.036
Shieh, P. I., Jeng, Y. C., & Tsai, M. P. (2012). Agent based infrastructure with RFID technology for autonomous shop floor control system. Advanced Materials Research, 341–342, 596–600. https://doi.org/10.4028/www.scientific.net/AMR.341-342.596
Tran, N. H., Park, H. S., Nguyen, Q. V., & Hoang, T. D. (2019). Development of a smart cyber-physical manufacturing system in the Industry 4.0 context. Applied Sciences (Switzerland), 9(16). https://doi.org/10.3390/app9163325
Tranfield, D., Denyer, D., & Smart, P. (2003). Towards a Methodology for Developing Evidence-Informed Management Knowledge by Means of Systematic Review. British Journal of Management, 14(2), 207–222. https://doi.org/10.1080/16258312.2014.11517339
Wang, B., Cao, Z., Yan, Y., Liu, W., & Wang, Z. (2011). Fundamental technology for RFID-based supervisory control of shop floor production system. International Journal of Advanced Manufacturing Technology, 57(9–12), 1123–1141. https://doi.org/10.1007/s00170-011-3358-7
Wang, C., Jiang, P., & Ding, K. (2017). A hybrid-data-on-tag-enabled decentralized control system for flexible smart workpiece manufacturing shop floors. Proceedings of the Institution of Mechanical Engineers, Part C: Journal of Mechanical Engineering Science, 231(4), 764–782. https://doi.org/10.1177/0954406215620452
Wang, C. N., Hsu, H. P., Fu, H. P., Phan, N. K. P., & Nguyen, V. T. (2022). Scheduling flexible flow shop in labeling companies to minimize the makespan. Computer Systems Science and Engineering, 40(1), 17–36. https://doi.org/10.32604/CSSE.2022.016992
Yao, X., Zhang, J., Li, Y., & Zhang, C. (2018). Towards flexible rfid event-driven integrated manufacturing for make-to-order production. International Journal of Computer Integrated Manufacturing, 31(3), 228–242. https://doi.org/10.1080/0951192X.2017.1407455
Zermane, H., & Drardja, A. (2022). Development of an efficient cement production monitoring system based on the improved random forest algorithm. International Journal of Advanced Manufacturing Technology, 1–16. https://doi.org/10.1007/s00170-022-08884-z
Zhang, Y., Huang, G. Q., Sun, S., & Yang, T. (2014). Multi-agent based real-time production scheduling method for radio frequency identification enabled ubiquitous shopfloor environment. Computers and Industrial Engineering, 76(1), 89–97. https://doi.org/10.1016/j.cie.2014.07.011
Zhang, Y., Qu, T., Ho, O. K., & Huang, G. Q. (2011). Agent-based Smart Gateway for RFID-enabled real-time wireless manufacturing. International Journal of Production Research, 49(5), 1337–1352. https://doi.org/10.1080/00207543.2010.518743
Zheng, Z., Zhang, K., & Gao, X. (2022). Human-cyber-physical system for production and operation decision optimization in smart steel plants. Science China Technological Sciences, 65(2), 247–260.
Zhong, R. Y., Huang, G. Q., Lan, S., Dai, Q. Y., Chen, X., & Zhang, T. (2015). A big data approach for logistics trajectory discovery from RFID-enabled production data. International Journal of Production Economics, 165, 260–272. https://doi.org/10.1016/j.ijpe.2015.02.014
Zhong, R. Y., Li, Z., Pang, L. Y., Pan, Y., Qu, T., & Huang, G. Q. (2013). RFID-enabled real-time advanced planning and scheduling shell for production decision making. International Journal of Computer Integrated Manufacturing, 26(7), 649–662. https://doi.org/10.1080/0951192X.2012.749532
Zhou, T., Tang, D., Zhu, H., & Zhang, Z. (2021). Multi-agent reinforcement learning for online scheduling in smart factories. Robotics and Computer-Integrated Manufacturing, 72(May), 102202. https://doi.org/10.1016/j.rcim.2021.102202
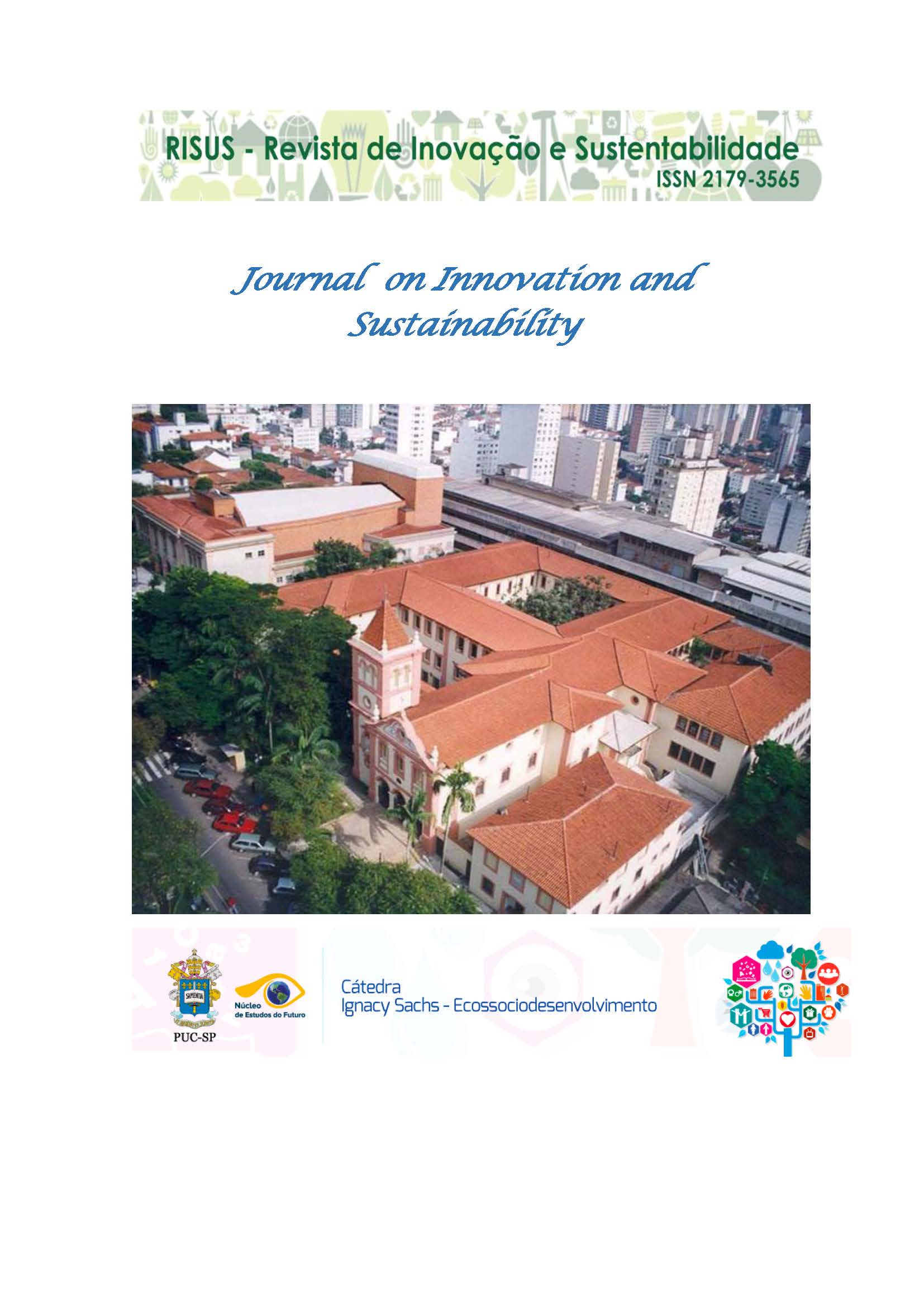
Descargas
Publicado
Número
Sección
Licencia
This Journal is licensed under a Creative Commons Attribution-Non Commercial-No Derivers 4.0 International license.
1.The author (s) authorize the publication of the article in the journal;
2.The author (s) warrant that the contribution is original and unpublished and is not in the process of being evaluated in other journal (s);
3. The journal is not responsible for the opinions, ideas and concepts emitted in the texts, as they are the sole responsibility of its author (s);
4. The editors are entitled to make textual adjustments and to adapt the articles to the standards of publication.